Build your topical authority with the help of the TF-IDF tool
In 2023, search engines look for topical relevance in search results, as opposed to the exact keyword match of the early web SEO. To help you do keyword research and on-page optimization in the age of semantic search, we added the TF-IDF algorithm to WebSite Auditor.
Using the TF-IDF technique, you will find numerous topical keywords and phrases to add to your pages — terms that will improve the topical relevance of your pages and make them rank better in Google search results.
To start using TF-IDF, all you need to do is download WebSite Auditor and jump right to the Page Audit module.
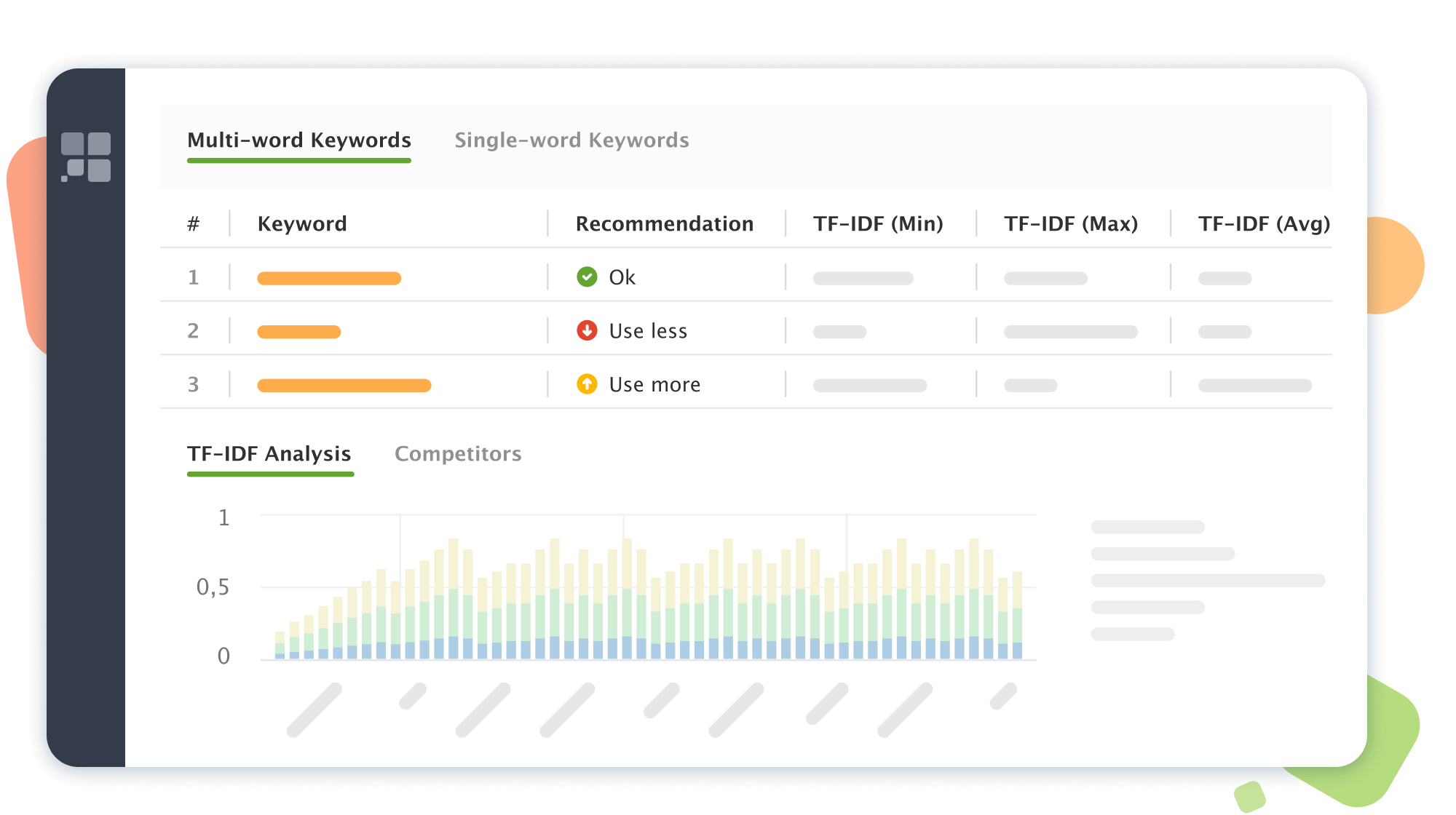
Improve your site SEO using TF-IDF analysis
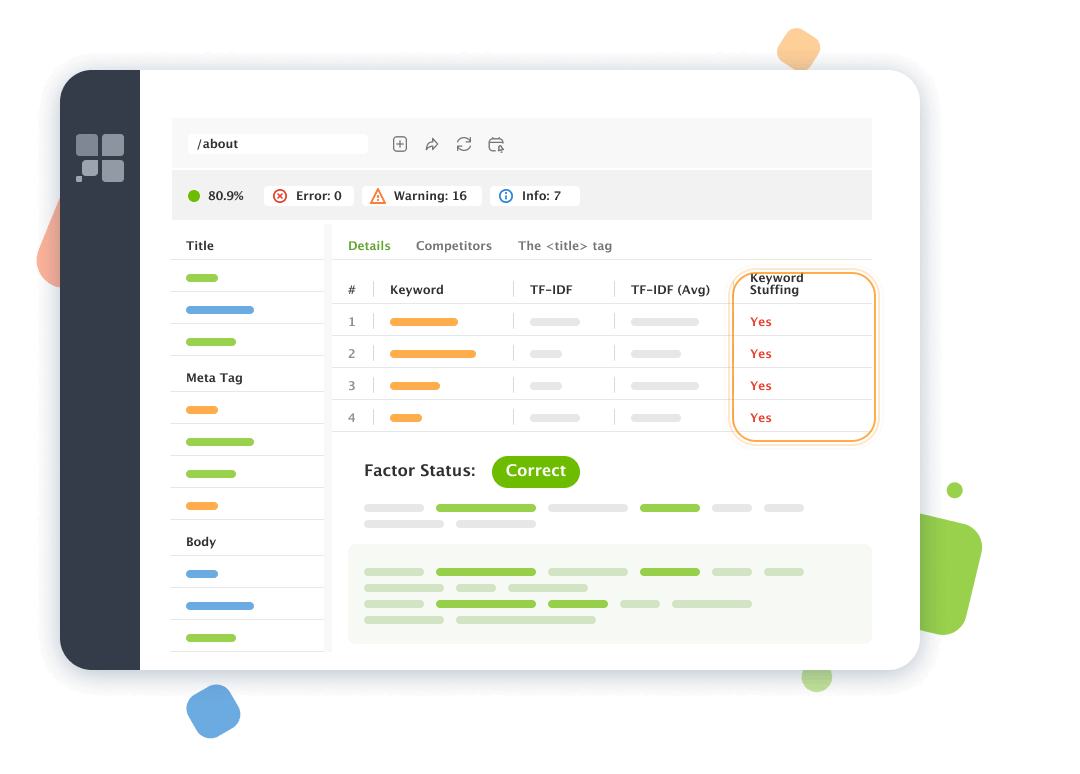
You may be surprised to find that you're overusing certain terms in your content, and not using enough of others.
Under the TF-IDF dashboard, look for the words and phrases with Use less or Use more recommendations to see how you can tweak your copy to improve relevance.
Discover the keywords and phrases that your top-ranking competitors are using — these terms can improve your page's topic relevance and help it rank better.
Switch between Single-word Keywords and Multi-word Keywords to look for separate words and phrases.
Look for the keywords with an Add recommendation — these are the terms most of your competitors use while you don't.
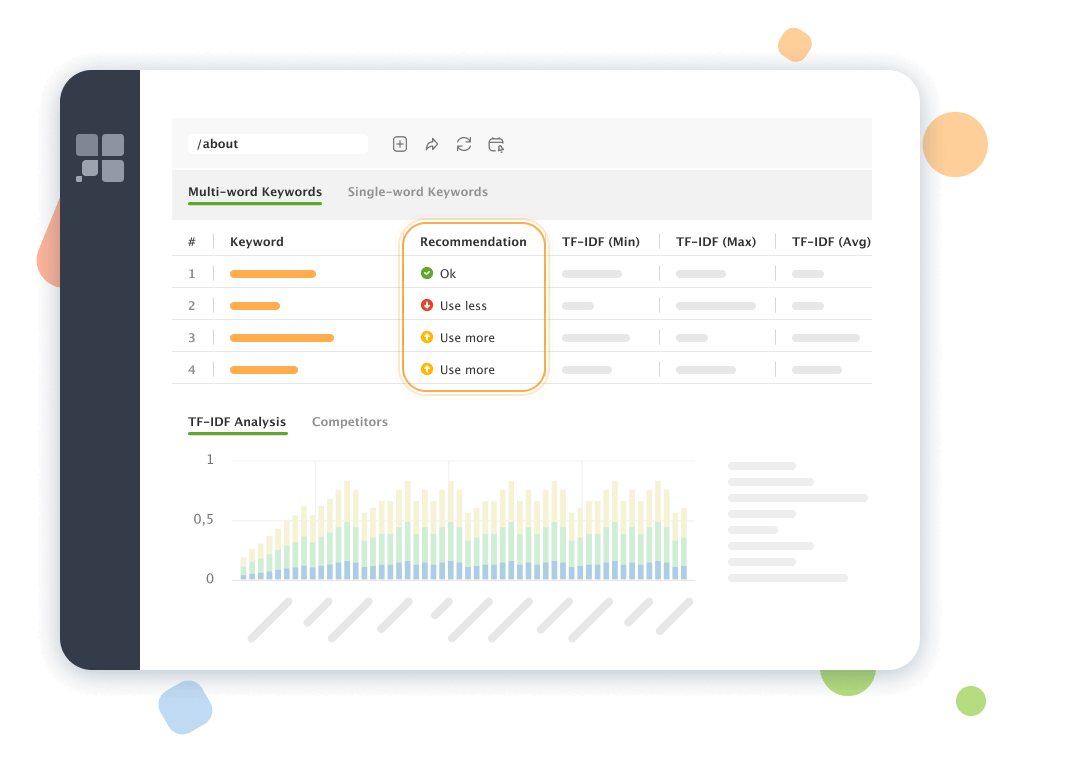
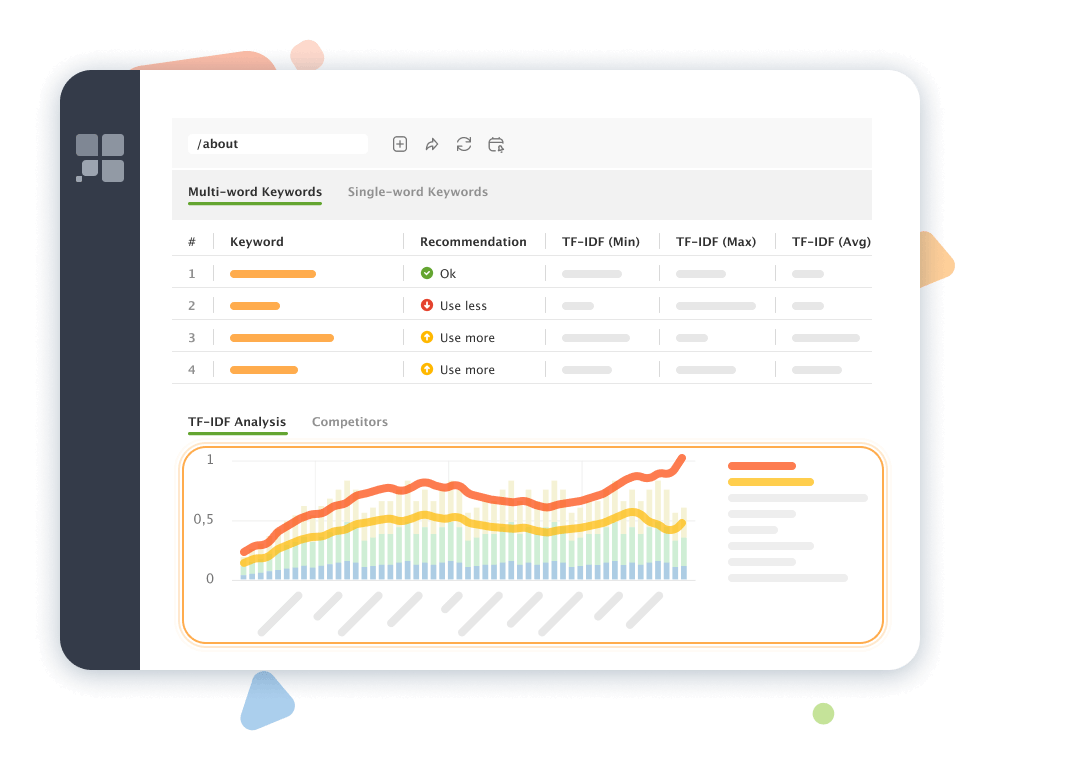
With the TF-IDF algorithm used to calculate content optimization factors, these metrics have become much more robust and reliable.
The tool can audit content of each URL, analyzing how well your page is optimized for your target keywords.
Now that you know which keywords you need to add, use more, or use less of, edit your content on the go right in the in-built Content Editor.
After you added the necessary changes, hit the Export the document to HTML down arrow to save the optimized version of your HTML to your computer.
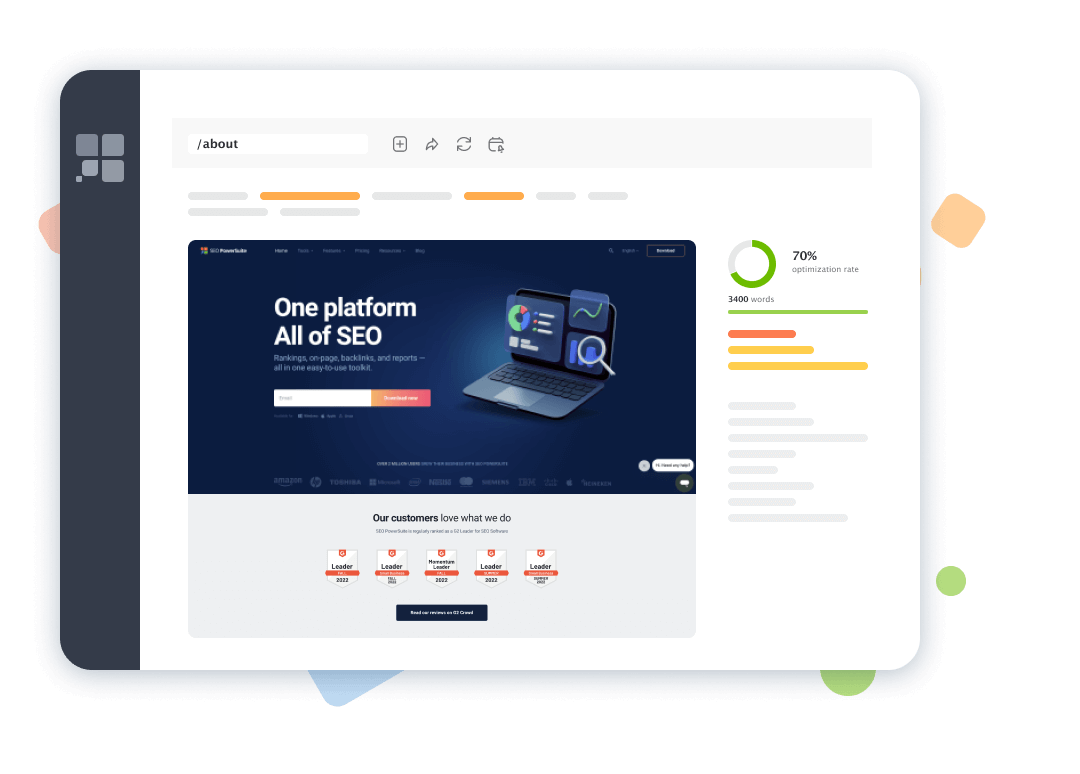
What is the TF-IDF technique in SEO?
TF-IDF ("term frequency — inverse document frequency") is an algorithm used to measure the importance of a certain keyword on a web page. Unlike keyword density, it doesn't just look at the number of times the term is used on the page, it also analyzes a larger set of pages and tries to determine how important this or that word is.
For example, in car repair, the term “tire repair” is likely more important than “turbocharged engine repair” — simply because every car has tires, and only a small number of cars have turbo engines. Because of that, the former is going to be used in a larger set of pages about this topic.
And this is exactly what the TF-IDF tool in WebSite Auditor will be able to catch! The TF-IDF formula is going to show you if your content is optimized adequately (as much as search engines expect them to appear, since Google has made TF-IDF part of its indexing). Looking at keyword usage stats of a large number of your competitors, the tool shows
Use WebSite Auditor's TF-IDF tool for keyword ideas
How to use the TF-IDF tool in WebSite Auditor
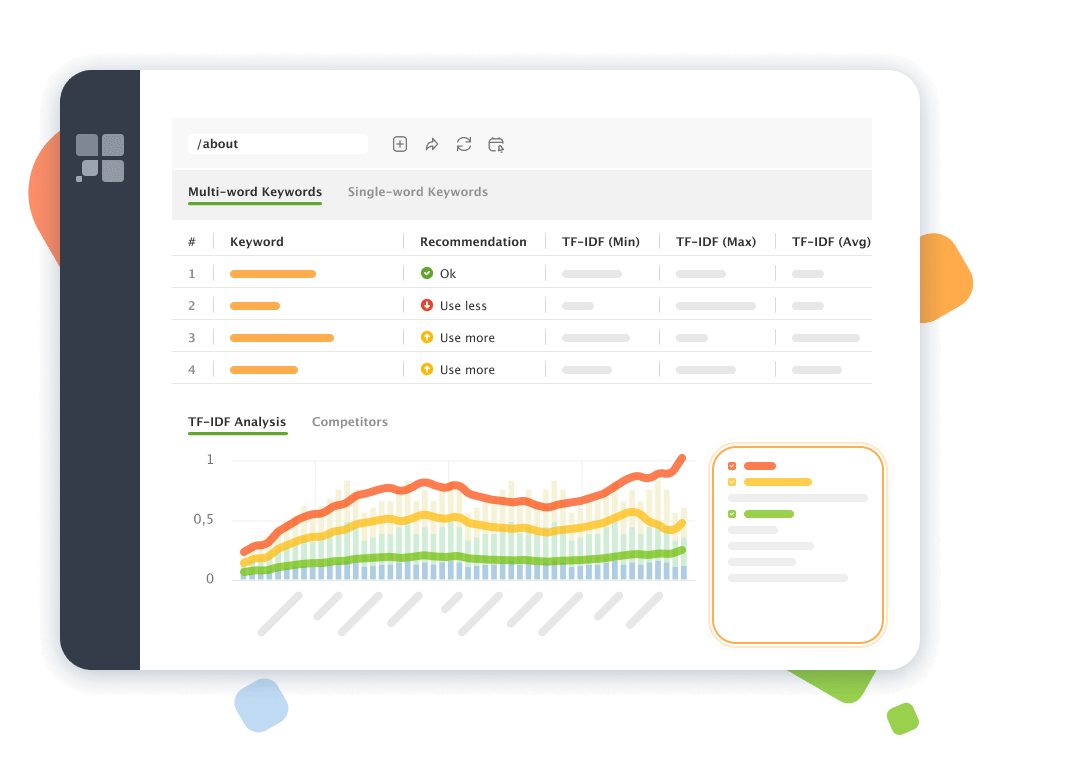
Under Page Audit > TF-IDF, you'll find the full list of terms and phrases associated with your target keyword based on your top-ranking competitors' content.
Here, you'll also get usage recommendations for specific terms and see how your keyword utilization compares to competitors' on the TF-IDF chart.
TF-IDF is also used to calculate optimization metrics under WebSite Auditor's Content Audit dashboard.
Unlike the old-school metrics like keyword density, TF-IDF will accurately determine if there are keyword stuffing or under-optimization issues in your content or any given page element.
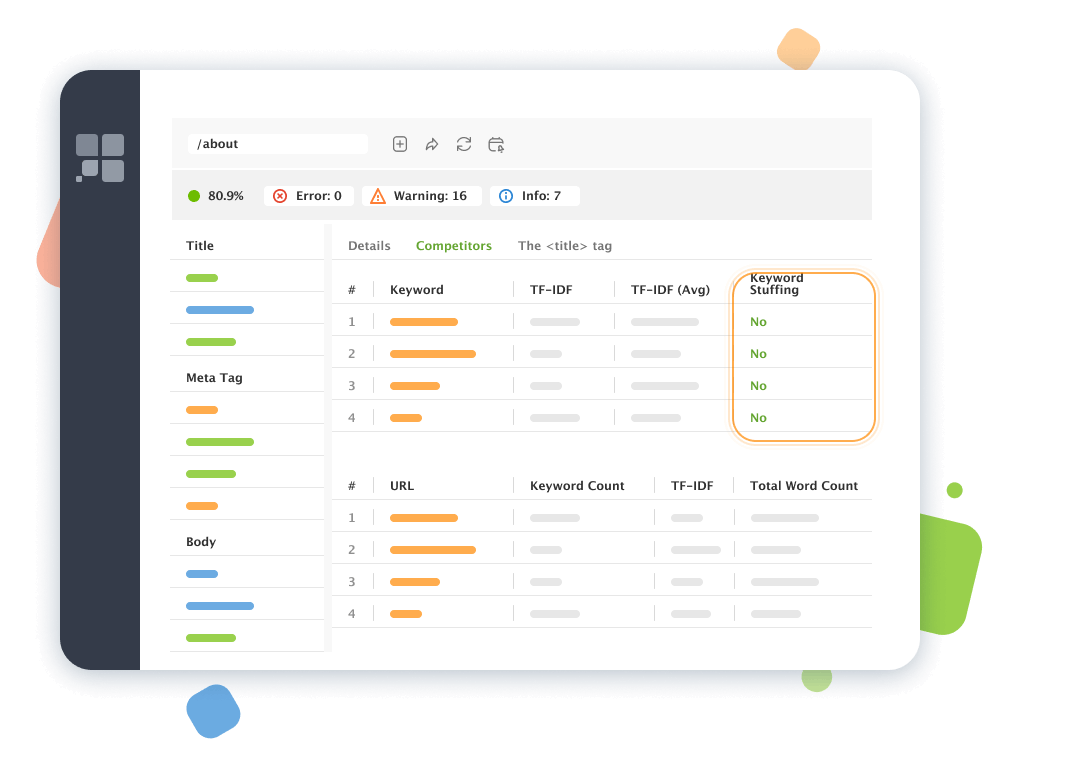
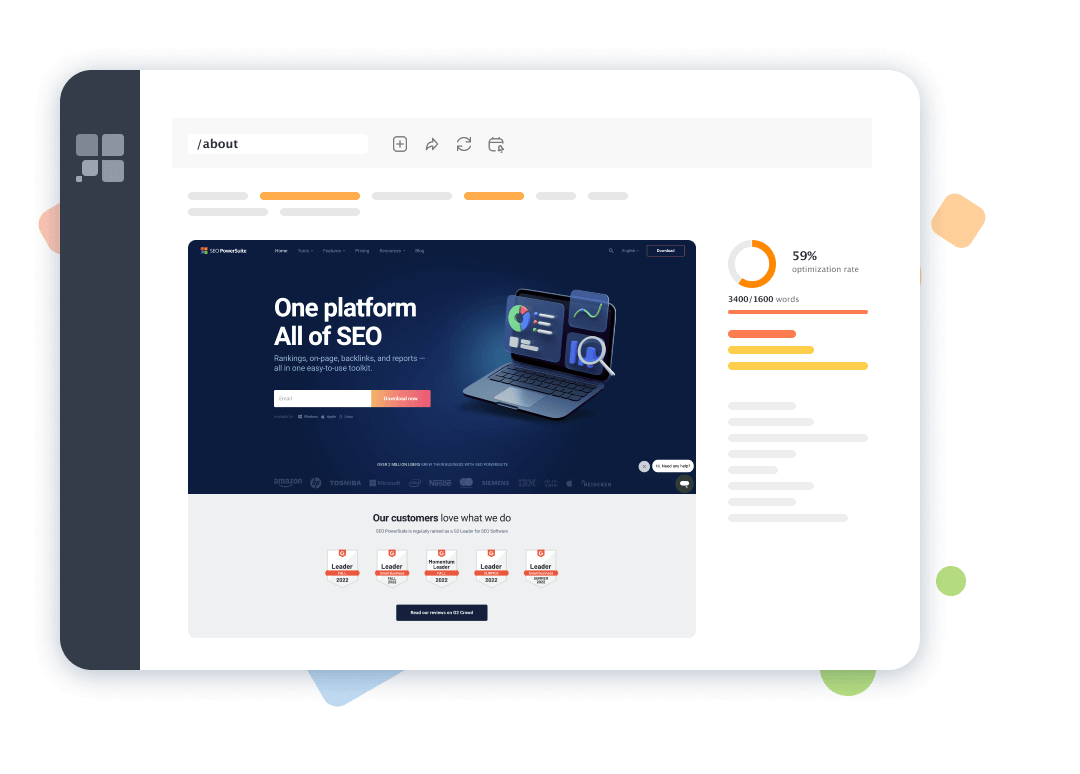
In Page Audit > Content Editor, your content optimization tips are now also based on TF-IDF analysis of the keyword usage stats of the top-ranking competitors.
This ensures more accurate optimization advice than ever before, perfectly tailored to your pages and keywords.
Smarter keyword ideas and optimization advice in the age of semantic search
Use the free TF-IDF tool for unlimited content ideas and optimization advice. Choose to upgrade to a Pro or Enterprise version any time you like to get access to agency features.